Introduction
Picture this: an executive, tasked with integrating AI into their organization, trying desperately to sort through the noise to figure out how all of this applies to their business. They’ve heard terms like generative AI and predictive AI, but what do those terms mean exactly? How can they be used? Where should one be used versus the other? Let’s give them a hand by explaining the core essence of each:
✍🏻 Generative AI
Is rooted in creativity, autonomously producing original content like text, art, or music. It generates.
📊 Predictive AI
Focuses on forecasting future outcomes based on existing data and guiding decision-making. It predicts.
Finding the Balance: Navigating Creativity and Data in Organizational Strategy
While that helps to frame up the conversation, it still leaves our exec wondering which is appropriate for their organization. It’s like standing at a crossroads with two signs pointing in opposite directions. On one side, there’s the flashy sign of creative innovation, promising excitement and big ideas. On the other, there’s the practical sign of data and analysis, offering stability and predictability. As they weigh their options, they realize that maybe the best path forward is a bit of both – a mix of creativity and crunching numbers – to steer the company towards success.
In this article, we’ll explore that idea. We’ll start with an explanation of both generative and predictive AI, briefly discussing their respective applications for businesses. We’ll next compare the two, giving guidance on when to choose one over the other. After that, we’ll take a look at where the real magic come in – using the two in tandem to produce novel, value-producing results. We’ll wrap up with some key business implications and considerations as you move forward on your AI journey, empowering you to act with confidence and drive innovation in your organization.
Generative AI: Fostering Creativity, Innovation, and Bespoke Experiences
Generative AI stands at the forefront of innovation, promising to revolutionize the way businesses approach creativity and content generation. At its core, generative AI harnesses sophisticated algorithms to learn from vast datasets, identify patterns, and generate new content autonomously. Unlike traditional AI systems that operate within predefined parameters, generative AI ventures into uncharted territory, quickly producing large volumes of original content that mimics or even transcends human creativity.
One of the most remarkable aspects of generative AI is its ability to foster innovation and exploration. By generating diverse and unconventional outputs, generative AI pushes the boundaries of creativity, inspiring new ideas and solutions that may have never been conceived otherwise. This creative potential empowers businesses to differentiate themselves in competitive markets, drive customer engagement, and fuel brand innovation.
Additional techniques can augment this unprecedented creativity to enable a powerful fusion of generative AI and factual data. Retrieval-augmented generation (RAG) offers a novel approach to content generation that combines generative models with knowledge retrieval capabilities. RAG has the ability to generate high-quality, contextually relevant content by leveraging pre-existing knowledge from vast repositories of information. This enables businesses to produce more accurate and informative content. RAG facilitates the creation of content that is tailored to specific user queries or preferences, enhancing both engagement and satisfaction.
The potential applications of generative AI span a wide array of industries, from agriculture and manufacturing to entertainment and marketing.
👨🏻🌾 In agriculture, generative AI
Enables personalized, timely, actionable communications between experts and growers to provide the best care for their crops.
🏭 In design and manufacturing
Generative AI revolutionizes product development, enabling rapid prototyping and optimization through iterative design processes.
🎨 In the realm of art and music
Generative AI algorithms compose symphonies, generate paintings, and even produce entire novels, blurring the lines between man and machine.
🪧 In marketing and advertising
Generative AI facilitates hyper-personalized content creation, allowing businesses to tailor their messaging to individual preferences and demographics.
In the next section, we’ll shift our focus to predictive AI and explore how it enables businesses to anticipate future trends and make informed decisions based on data-driven insights.
Predictive AI: Optimizing Decision-Making
The ability to anticipate future trends and outcomes is a critical business capability to stay ahead of the curve. Predictive artificial intelligence (AI) is a powerful tool in this pursuit, leveraging advanced algorithms and machine learning techniques to analyze historical data and forecast future events with remarkable accuracy.
Simply put, predictive AI operates on the principle of pattern recognition, uncovering hidden insights and trends to predict future outcomes. By identifying correlations and causations, predictive AI empowers businesses to make informed decisions, mitigate risks, and capitalize on emerging opportunities.
The applications of predictive AI span a multitude of industries and use cases.
- 👨🏻🌾 In agriculture, predictive AI models predict pest outbreaks by analyzing environmental factors and past infestations, allowing farmers to implement targeted pest management strategies and minimize crop damage.
- 🏥 In healthcare, predictive AI aids in disease diagnosis and treatment planning by analyzing patient data and predicting potential health outcomes.
- 🏦 In finance, predictive AI models analyze market data to forecast stock prices, detect fraudulent transactions, and optimize investment strategies.
- 💼 In staffing, predictive AI analyzes job market trends, candidate behavior, and historical hiring data to forecast future talent demand, enabling staffing agencies to proactively source, train, and place candidates to meet evolving industry needs.
One of the key advantages of predictive AI
Is its ability to continuously learn and adapt in real-time. As new data becomes available, predictive AI models adjust their predictions, ensuring that decision-makers are equipped with the most up-to-date insights. This iterative learning process enhances the accuracy and reliability of predictions over time, enabling businesses to make proactive decisions and stay ahead of the competition.
However, the effectiveness of predictive AI hinges on the quality and availability of data. To derive meaningful insights, businesses must ensure access to comprehensive and high-quality datasets, free from biases and inaccuracies. Additionally, organizations must invest in robust data infrastructure and analytics capabilities to extract actionable insights from the data deluge. As the realm of AI rapidly expands, it’s essential for businesses to embrace a data-driven mindset and leverage predictive analytics to drive strategic decision-making.
Now that you understand the fundamentals of both approaches, we’ll next explore the differences between generative and predictive AI, guiding you toward the approach that best meets your business objectives.
Contrasting Generative and Predictive AI
While both generative AI and predictive AI harness the power of artificial intelligence, they diverge in their objectives, methodologies, and applications. Understanding the distinctions between these two approaches is essential for businesses seeking to leverage AI effectively and strategically.
✍🏻 Generative AI focuses on creativity and innovation. It excels at generating new, original content autonomously, whether it’s in the form of images, music, text, or even entire narratives. Gen AI is often used where innovation and originality are paramount, pushing the boundaries of creativity and inspiring new ideas that may not have been conceivable through traditional means. It can fuse factual information with creativity to generate personalized, bespoke experiences for customers.
📊 Predictive AI focuses on forecasting future outcomes based on historical data patterns. It analyzes vast datasets to identify trends, correlations, and causations, enabling businesses to anticipate customer behaviors, forecast market trends, and optimize operational processes with precision and efficiency. It facilitates strategic decision-making and risk management and is utilized when data-driven insights are instrumental in driving business outcomes.
Here are some questions you should consider when trying to decide if generative AI, predictive AI, or a more traditional approach is the correct choice to achieve your goal:
- What is the goal?
- If the goal involves complex patterns or requires creativity, consider generative AI.
- If the goal is data-driven and requires predictions, consider predictive AI.
- What data is available?
- If there is limited or no historical data available, consider generative AI.
- If there is ample structured data available, consider predictive AI.
- If the data is qualitative or unstructured, you’ll need to consider other factors like risk tolerance and time constraints.
- Is interpretability & explainability important?
- Interpretability refers to the transparency of an AI model – being able to interpret the inner workings of why and how a model generates outputs.
- Explainability is the ability to explain a model’s behavior in human terms.
- If explainability isn’t needed, or is outweighed by the need for accurate predictions, consider predictive AI.
- If understanding the underlying process is less important than results, generative AI may be an alternative option.
- What are the time constraints?
- If you need quick decision-making based on existing knowledge, traditional means may suffice.
- If there’s time for model training and validation, predictive AI becomes viable.
- If time permits exploration of novel solutions, generative AI may be worth considering.
- What is the tolerance for risk and uncertainty?
- If risk aversion is paramount and conservative approaches are preferred, consider traditional means are likely the safest route.
- If there’s tolerance for uncertainty and a willingness to experiment, predictive AI could provide valuable insights.
- If embracing uncertainty and exploring diverse possibilities is desirable, generative AI may lead to more innovative solutions.
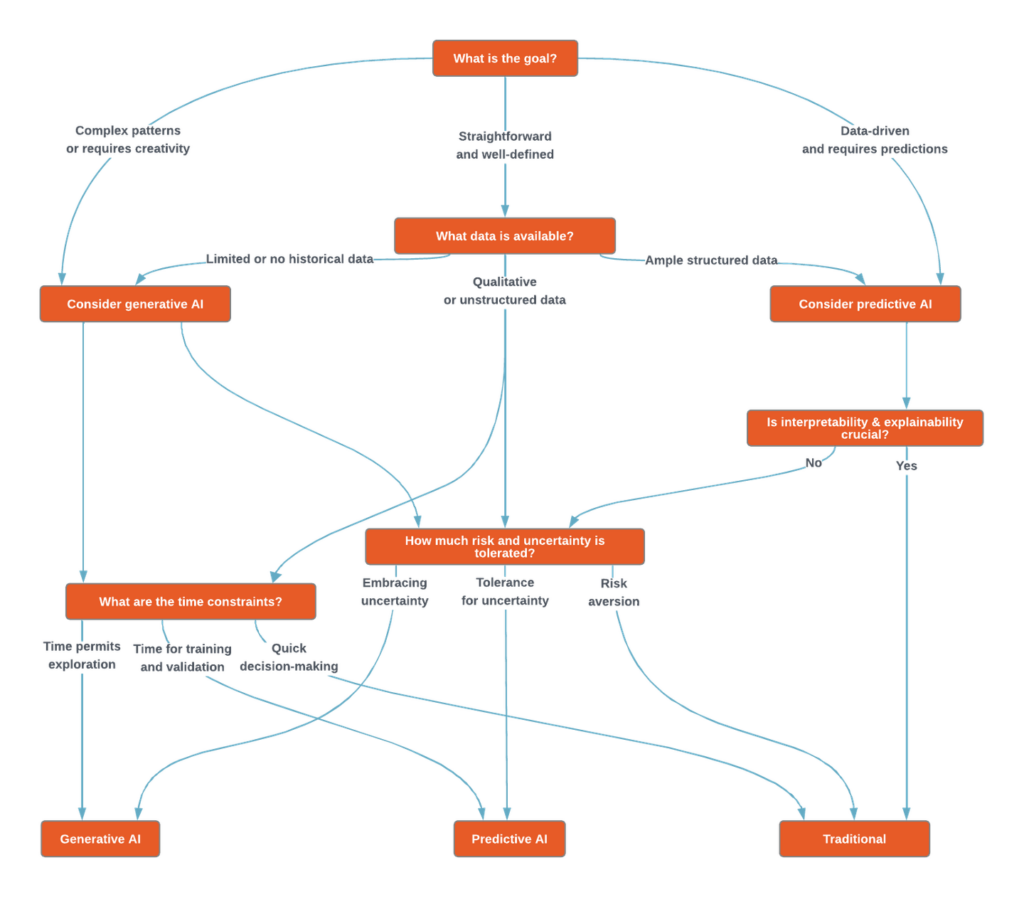
Strategic Considerations: Navigating AI Costs and Implementation Strategies
An additional consideration with high importance but without a clear cut answer is cost. The number of AI offerings and their pricing is rapidly evolving, leading to a wide range of potential costs when implementing an AI solution. In general, you can expect the initial cost of building predictive models from scratch to be the most expensive and variable, while working from pre-built models or using Software as a Service (SaaS) offerings is usually cheaper upfront and has more predictable ongoing costs.
While this is not an exhaustive list of questions, it will help to place guardrails around your decision and determine if a choice is viable. Keep in mind, however, that your options are not mutually exclusive. In the next section, we’ll explore combining approaches to further hone your competitive edge.
Complementary Capabilities
Despite their differences, generative and predictive AI can complement each other synergistically. By combining generative AI’s creativity with predictive AI’s foresight, businesses can unlock new possibilities and drive innovation. Let’s look at several use cases across different industries where using both approaches unlocks additional value:
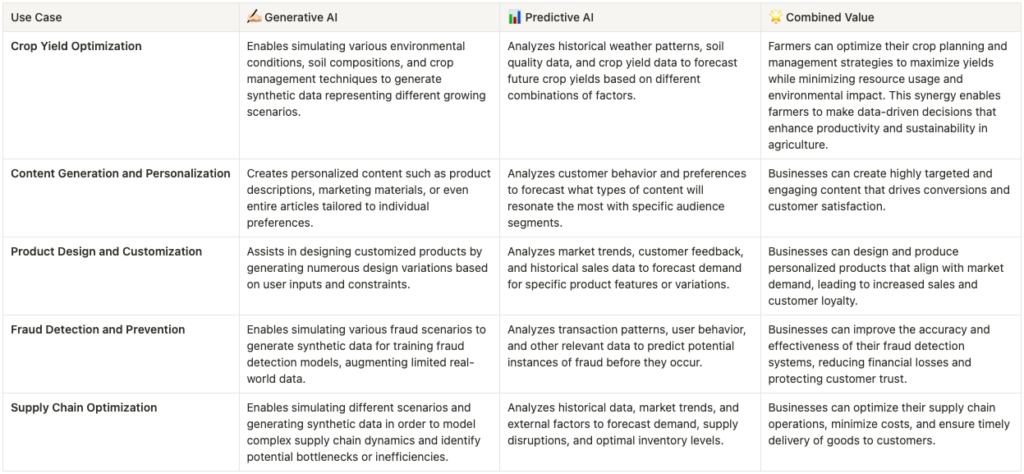
It’s important to remember that while both generative and predictive AI have their own unique strengths and limitations, working together they can unlock new capabilities and harness the full potential of AI. In the next section, we’ll look at key considerations to bringing these technologies to fruition in your organization.
Strategic Implications for Executive Decision-Makers
Harnessing the potential of generative and predictive AI requires more than just understanding them technically. It demands a strategic approach that aligns AI initiatives with organizational goals, fosters innovation, and addresses ethical considerations. If you’re ready to dip your toes into AI, here are a few key areas to consider as part of your strategy:
1. Aligning AI Strategies with Organizational Objectives:
- Recognize AI as a strategic enabler and integrate it into long-term business plans
- Define clear objectives and key performance indicators (KPIs) to measure the impact of AI initiatives on business outcomes
- Collaborate across departments to ensure alignment between AI initiatives and broader organizational goals
2. Considering Speed to Market
- Recognize that there is an AI “Gold Rush” happening, and speed to market will be critical as competitors integrate AI into their strategies and products
- Determine whether existing resources have the required skillsets in a rapidly evolving space to quickly identify and implement AI solutions that provide value
- Evaluate the use of Software as a Service (SaaS) offerings, pre-built models, and skilled partners to kickstart your AI initiatives and get to market quicker
3. Defining Talent Acquisition and Upskilling Initiatives:
- Invest in talent acquisition strategies to attract skilled AI professionals and data scientists
- Provide ongoing training and development opportunities to upskill existing employees in AI-related competencies
- Foster a culture of continuous learning and innovation to drive AI adoption and integration across the organization
4. Leveraging AI for Innovation and Competitiveness:
- Encourage experimentation and exploration of AI technologies to uncover new opportunities for innovation
- Foster cross-functional collaboration and knowledge sharing to leverage AI insights across departments
- Stay abreast of emerging trends and advancements in AI to maintain a competitive edge in the market
5. Navigating Ethical and Regulatory Concerns:
- Establish ethical guidelines and frameworks to govern the responsible use of AI
- Address biases in AI algorithms and datasets to ensure fairness and equity
- Ensure legal requirements (e.g., explainability compliance in some sectors or regions) are frequently monitored, met, and considered for future initiatives
- Safeguard consumer privacy and data protection through robust security measures and compliance with regulatory requirements
By embracing a strategic approach to AI adoption, executives can ensure AI initiatives are led with vision, foresight, and care.
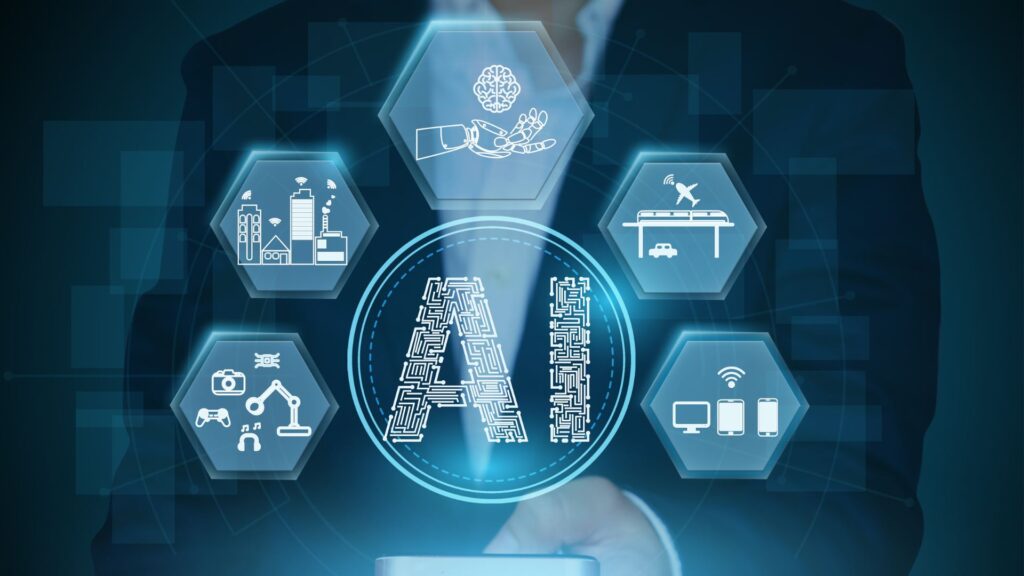
Conclusion
As we conclude our exploration of generative and predictive AI, it’s evident that these technologies hold immense potential to reshape the future of business. From fostering creativity and innovation to enabling data-driven decision-making, AI will quickly become an integral part of how organizations operate, compete, and thrive. Advancements in AI continue to happen an incredible pace, increasing the breadth of use cases that it can address and making it even more critical to understand, plan for, and adopt as soon as possible. Business decision makers play a pivotal role in this adoption. By understanding the nuances of generative and predictive AI and adopting a strategic approach to their implementation, you can unlock new opportunities for growth and success and blaze a trail into the new AI-enabled world.